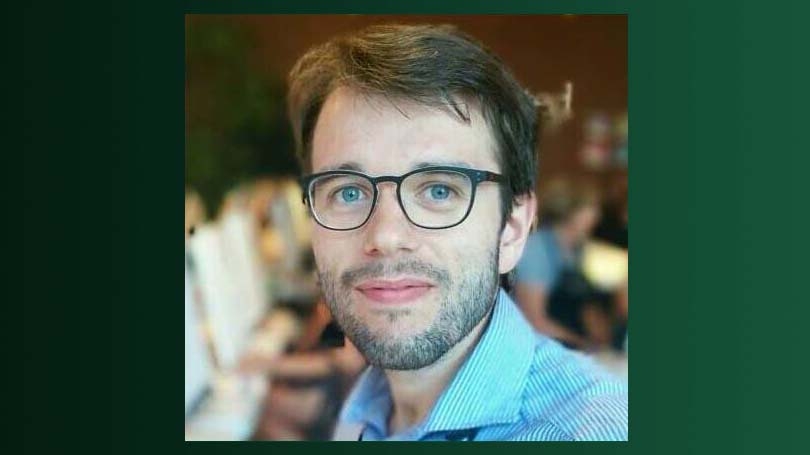
Thanks to the GRAD Alumni Research Award, Psychological and Brain Sciences PhD candidate Matteo Visconti was able to amass data on facial recognition processes much faster
Faces convey an astounding quantity of information that facilitates social interactions. Through faces, we can distinguish and recognize identities, accurately detect social cues that signal emotion and direction of attention, and we can make categorical judgments of gender, age, and ethnicity. The multiple streams of information we gather from faces are essential to navigate our complex social lives. How our brain represents this information is the focus of my Ph.D. research in Dr. M. Ida Gobbini’s lab in Dartmouth’s Psychological and Brain Sciences department.
Neuroimaging studies have shown that a distributed network of brain areas acts in concert to process faces. The current model for this network posits a distinction between visual processing of the unchanging structural appearance of a face and the changeable aspects that signal socially relevant information, such as expression or direction of eye gaze (Haxby et al., 2000). While this model has influenced almost two decades of research in cognitive neuroscience, the exact role of the areas of this network—and whether other areas should be included in this model—is still under active investigation. Much of the previous research in the field has used a limited set of static images to investigate this complex network. The use of static images makes it easier to control for possible confounding factors such as color or brightness; however, this approach makes experiments less ecologically valid. Everyday visual input is dynamic, visually cluttered, and more complex than standard lab experiments.
Following recent advances in experimental design for neuroimaging studies, Dr. Gobbini and I wanted to investigate the distributed functional network for face processing with stimuli that are closer to our everyday life in terms of richness of visual stimulation. We collected hundreds of video clips obtained from YouTube depicting people from a variety of ethnicities, ages, and gender. The individuals were talking, looking around, and displaying different emotions. Participants in our experiment watched these video clips, while we were sampling their brain activity using functional Magnetic Resonance Imaging (fMRI). With these data at hand, we are using advanced computational models to link the content of the clips to neural activity, teasing apart where in the brain the different attributes of a face (e.g., identity, age, ethnicity, head angle, gender, etc.) are processed, and how the different components of the network for face processing interact to extract complex information such as identity.
In this type of analysis, it is essential to describe and label each individual stimulus to map its content to brain activity. Because of the number of stimuli used in this experiment, manual annotation by a single person is cumbersome and impractical. Thanks to the funds from the Alumni Research Award, I was able to recruit participants using Amazon MechanicalTurk’s service. For example, in order to collect ratings of the video clips that were used in one of my experiments, I recruited 150 people, which allowed me to have each of the hundreds of video clips labeled by at least 10 different people in less than a day. Had I not received the Alumni Research Award, this step would have taken months, becoming a major bottleneck for this project.
We are going to use these labels, together with features extracted from computer vision algorithms, as inputs to predict brain activity related to face processing. We expect to better characterize the function of the different components of the network for face processing by exploiting these naturalistic, dynamic stimuli. Such a detailed predictive model will also be helpful to investigate individual differences in the spectrum of face processing abilities. The model will advance our understanding of how our visual system builds up stable and noise-resistant representations of familiar individuals.