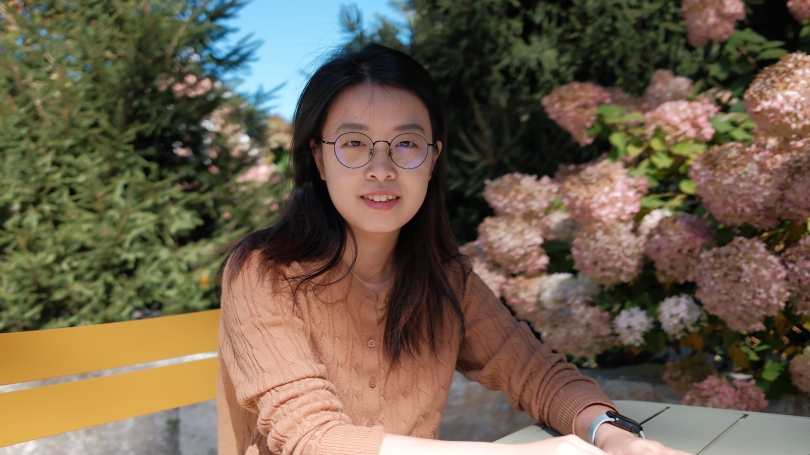
Yuxin Wang created a dataset that tests AI models' ability to identify manipulative language in conversations. (Photo credit: Yanjun Cui, Guarini)
Like most things in our lives today, interpersonal conversations have also gone digital.
"With private conversations moving to messaging apps and social media, there are increasing instances of people enduring mental and emotional manipulation online," says Yuxin Wang, Guarini, a second-year computer science PhD student, who works with Soroush Vosoughi, assistant professor of computer science and Saeed Hassanpour, professor of biomedical sata science and epidemiology and director of the Center for Precision Health and Artificial Intelligence.
Wang defines mental manipulation, such as gaslighting, as a form of verbal abuse that deliberately aims to control or influence someone's thoughts for personal benefit. Threats of career sabotage from an employer or supervisor, or emotional blackmail in toxic relationships, are common examples.
Because such language is implicit and context dependent, recognizing manipulative language can be very challenging for large language models, Wang says. These models power a quickly rising number of applications that we use every day to communicate as well as consume and create content.
To address this gap, Wang and her collaborators compiled a new dataset of conversations that showcase or highlight manipulation in conversations and used the dataset to analyze how effective state-of-the-art AI models are at identifying manipulative content.
The results of their study were presented at the annual meeting of the Association for Computational Linguistics in August.
The MentalManip dataset contains 4000 sets of fictional dialogues between a pair of characters extracted from movie scripts from the Cornell Movie Dialogs Corpus. They used two strategies to filter the data source and find dialogues with elements of manipulation.
The first was to manually compile a list of 175 key phrases that frequently occur in mental manipulation language such as "You are too sensitive" or "I know your manager." Dialogues in the source data were combed for matches to these phrases. The second method was to train a model to distinguish dialogues that are potentially manipulative through supervised learning.
The researchers then tested some well-known LLMs including OpenAI's GPT-4 and Meta's Llama-2. The models were tasked to identify whether a dialogue the researchers presented contained elements of manipulation.
A second experiment challenged models to identify which among three conversations contained manipulative language after they were shown some examples. Finally, the models were fine-tuned using labelled examples of manipulative language from the new dataset before testing their ability to identify manipulation.
The researchers found that the models were not equal to the task of categorizing manipulative content in all three instances.
The models, especially smaller LLMs, tend to identify general toxicity and foul language as manipulation, a sign of their undue oversensitivity. Their overall performance in detecting mental manipulation was unsatisfactory and did not improve with fine-tuning the models on existing relevant mental health or toxicity detection datasets. An analysis of the sentences in the conversations revealed that they are semantically indistinguishable, likely a contributing factor hindering the models' performance.
Wang hopes that their dataset and preliminary results will inspire more research on this topic. LLM models trained to reliably recognize manipulation could be a valuable tool for early intervention, warning victims that the other party is trying to manipulate them, says Wang.
Recognizing manipulative intent, especially when it is implicit, requires a level of social intelligence that current AI systems lack, according to Vosoughi.
"Our work shows that while large language models are becoming increasingly sophisticated, they still struggle to grasp the subtleties of manipulation in human dialogue," Vosoughi says. "This underscores the need for more targeted datasets and methods to effectively detect these nuanced forms of abuse."